Navigating the Costs of AI in Healthcare: Understanding Azure and Nebula Pricing
The adoption of Artificial Intelligence (AI) in healthcare has been accelerating, offering unprecedented opportunities for enhancing patient care, improving diagnostic accuracy, and streamlining operations. However, as healthcare organizations increasingly integrate AI technologies into their operations, understanding the associated costs becomes crucial. Azure, powered by Microsoft, and the Nebula platform, offer AI solutions with pricing based on actual usage. This blog explores the contributing factors to the cost of AI in healthcare and provides insights into managing expenses while maximizing the benefits of these technologies.
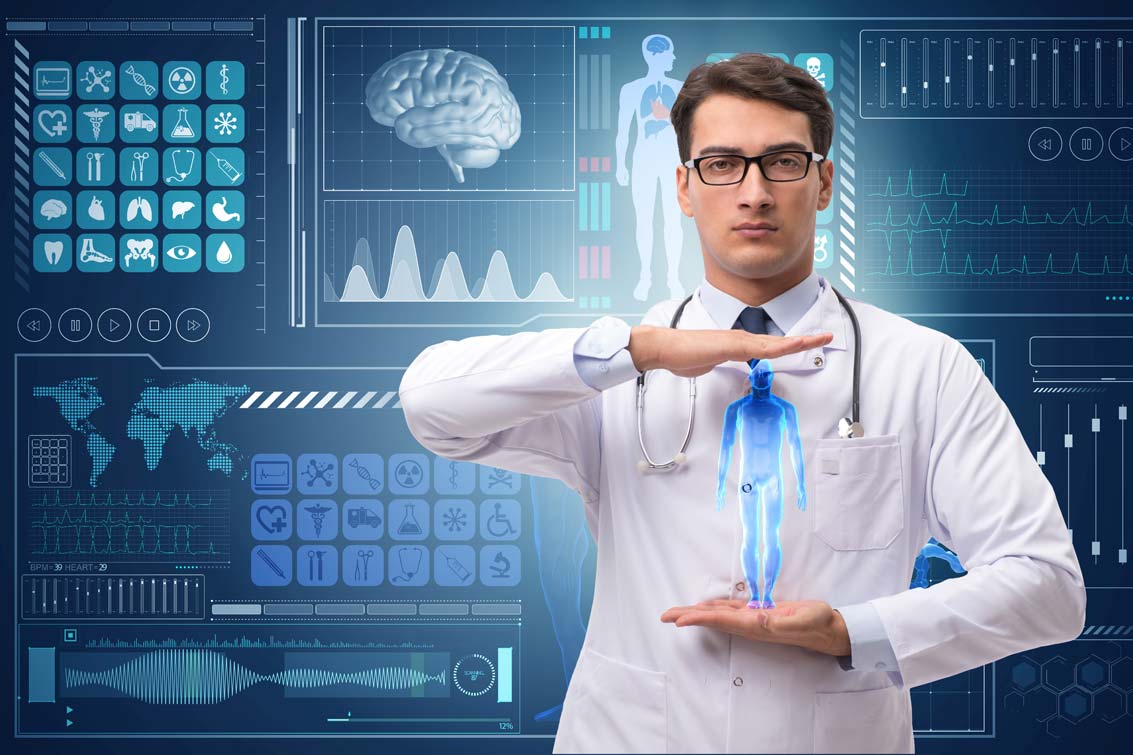
Azure Cost Based on Actual Usage
The Azure platform operates on a pay-as-you-go pricing model, which means that healthcare organizations are billed based on their actual usage of AI services. This model offers flexibility and scalability, allowing organizations to adjust their usage based on their needs without incurring unnecessary costs. When using AI through the Nebula platform, this cost model is passed directly onto the healthcare organizations, ensuring transparency and control over AI expenditures.
Contributing Factors to AI Costs
Several factors contribute to the cost of utilizing AI in healthcare through platforms like Azure and Nebula. Understanding these factors can help organizations budget effectively and make informed decisions about their AI strategies.
Number of Studies Sent to the Model
One of the primary cost drivers is the number of studies or datasets sent to the AI model for analysis. Each study sent incurs a cost, so the volume of data processed directly impacts the overall expense. Healthcare organizations should consider the necessity and value of each study submitted to optimize costs.
Length of Impressions Sent to the Model
The length of the impressions or the detailed information sent along with the studies for AI analysis also affects the cost. More extensive impressions may require more processing power and time, leading to higher charges. Organizations should aim to send concise, relevant impressions to manage costs effectively.
Generated Response Length
The length of the response generated by the AI model can also influence the cost. More detailed responses may incur higher costs due to the increased computational resources required. Healthcare organizations must balance the need for detailed insights with the associated costs to ensure cost-effective AI use.
Expected Cost per Study
On average, the expected cost for sending a study to the AI model through the Nebula platform is approximately $0.003. This cost estimate provides a baseline for healthcare organizations to budget their AI expenditures. However, actual costs may vary based on the factors mentioned above and the specific usage patterns of each organization.
Managing AI Costs in Healthcare
To effectively manage AI costs while leveraging its benefits, healthcare organizations should:
- Prioritize High-Value AI Applications: Focus on implementing AI in areas that offer the highest potential for improving patient care and operational efficiency.
- Monitor AI Usage: Regularly review AI usage patterns and costs to identify opportunities for optimization.
- Educate Staff: Ensure that all users understand the cost implications of their AI usage and are trained to use AI technologies efficiently.
- Leverage Predictive Analytics: Use AI itself to predict and manage the costs associated with its use, optimizing resource allocation.
Conclusion
The integration of AI in healthcare presents a promising avenue for enhancing various aspects of patient care and operational efficiency. While the cost of AI is an important consideration, the Azure and Nebula platforms offer flexible, usage-based pricing that allows healthcare organizations to manage their expenses effectively. By understanding the contributing factors to AI costs and adopting strategic approaches to AI implementation, healthcare organizations can maximize the benefits of AI technologies within their budgetary constraints.